Scalable Image-based Indoor Scene Rendering
with Reflections
Abstract
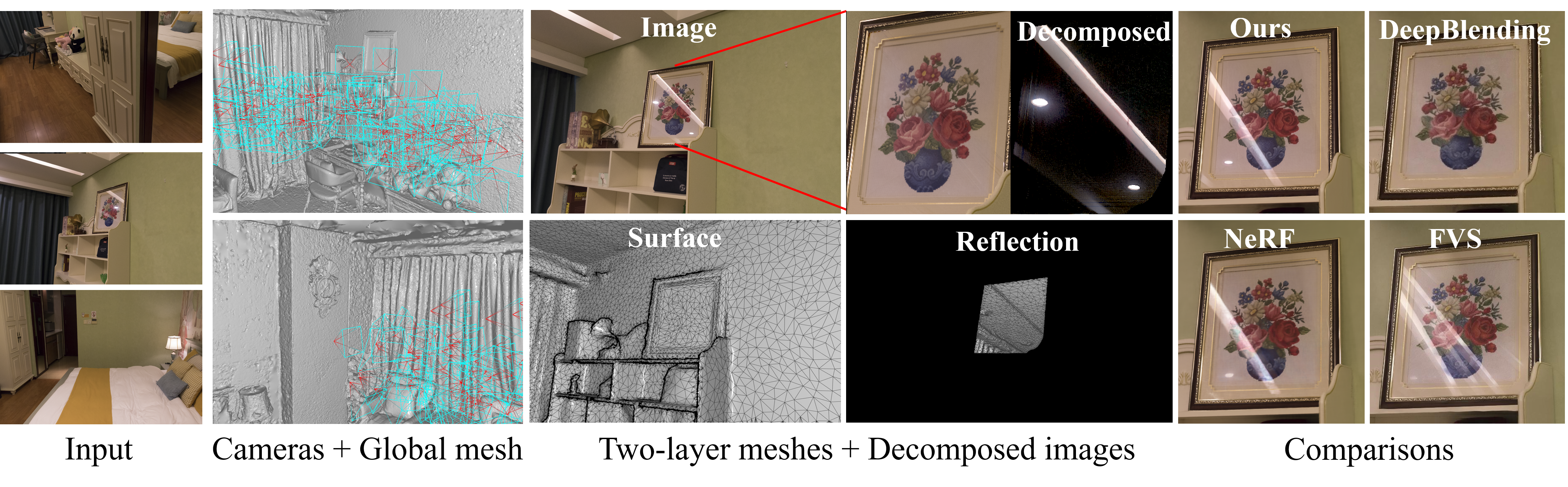
This paper proposes a novel scalable image-based rendering (IBR) pipeline for indoor scenes with reflections. We make substantial progress towards three sub-problems in IBR, namely, depth and reflection reconstruction, view selection for temporally coherent view-warping, and smooth rendering refinements. First, we introduce a global-mesh-guided alternating optimization algorithm that robustly extracts a two-layer geometric representation. The front and back layers encode the RGB-D reconstruction and the reflection reconstruction, respectively. This representation minimizes the image composition error under novel views, enabling accurate renderings of reflections. Second, we introduce a novel approach to select adjacent views and compute blending weights for smooth and temporal coherent renderings. The third contribution is a supersampling network with a motion vector rectification module that refines the rendering results to improve the final output's temporal coherence. These three contributions together lead to a novel system that produces highly realistic rendering results with various reflections. The rendering quality outperforms state-of-the-art IBR or neural rendering algorithms considerably.
Full Video
Citation
@inproceedings{xu2021scalable, title={Scalable Image-based Indoor Scene Rendering}, author={Xu, Jiamin and Wu, Xiuchao and Zhu, Zihan and Huang, Qixing and Yang, Yin and Bao, Hujun and Xu, Weiwei}, booktitle={ACM TOG}, year={2021} }